Every February, two teams from the National Football League (NFL) battle it out in the biggest single-event championship game in modern sports, the Super Bowl. With so much hype and attention placed on this one game, the details surrounding everything from who sang the national anthem to what television show aired afterwards have been documented in full since the very first game in 1967.
When building datasets within GIS, it's quite common to have missing or unknown values, incomplete entries and even data errors. Many GIS professionals envision themselves building maps and apps with a perfectly functioning geodatabase or feature layer only to discover gaps, mistakes and the dreaded <null> wreaking havoc across their desktops and web browsers.
Exceptions in GIS data are not always obvious to users who come from non-geospatial backgrounds. Sometimes explaining how a map or app didn’t turn out the way they were hoping for can be a challenge if users are not familiar with the concept of having complete data within an attribute table. I've found myself in a few situations where my descriptions about how much better a map or app would be with more complete attributes, or a few additional fields in the table, were met with blank stares and silence.
Referencing a complete dataset on a simple subject can help introduce these concepts to your non-geospatial audience members. I found success using historical data from the Super Bowl to show what cities the big game has been played in, including all the details as well as the promotional logo used each year. I simply composed a table by year and copied in all the relevant attributes I wanted to include from the many Super Bowl lists available on Wikipedia:
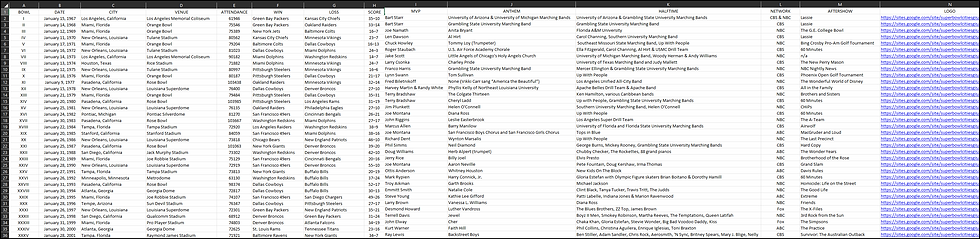
I excluded anything that would make the final dataset have gaps or fields that might be unclear. For example, the list of counterprogramming does not begin until 1992, so this would create a gap from 1967 to 1991 with no values. Furthermore, what network would I include, or would I make multiple fields to include multiple networks? The best choice here was to exclude it.
The resulting dataset was very easy to work with in the context of web GIS. I was able to load it as a CSV file into a web map on ArcGIS Online, using the cities as a locating field. From there, I could format my map pop-ups, compose my symbology, and package the result into a web app for the public to see:
Try using simple examples unrelated to whatever more complex or discipline-specific problem you might be trying to solve if you find yourself not getting through to a colleague or customer who typically doesn’t think spatially. Having real datasets to reference that meet your vision of what would work within a given map or app will only help your cause.
Comments